I appropriated, and over-simplified, the title of this blog from a recent article, Archaeological Analysis in the Information Age: Guidelines for Maximizing the Reach, Comprehensiveness, and Longevity of Data by Kansa et al. 2020, because it’s so true.
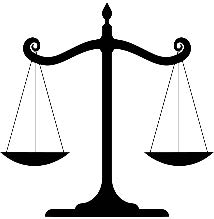
As part of the 2020 Kansa et al. article, which lays out a series of guidelines to improve “data management, documentation, and publishing practices so that primary data can be more efficiently discovered, understood, aggregated, and synthesized by wider research communities” (specifically in archaeology), the authors state, “…we strongly emphasize the importance of viewing data as a first-class research outcome that is as important as, if not more important than, the interpretive publications that result from their analysis.” I personally interpret this to mean that the data you produce are a more valuable contribution to your discipline than are many of your interpretations. Though this may sting a little for some to hear, I’m quite content with this idea. Science is bigger than me and my little sphere of knowledge and expertise, and I’m good with that.
As much as any academic is ever truly pleased with the final manuscript that’s been accepted to a peer-reviewed journal, there’s a good chance that you know that the results and conclusions drawn from that study are not even close to being 100% correct; especially in archaeology. We attempt to reconstruct past human behavior from an incomplete archaeological record, so the conclusions we draw about an event that created a biface or how North America was initially colonized can never be completely accurate. We know that there are flaws in our data (taphonomic and spatial bias), problems with the way statistical tests are interpreted, and even incongruences with the theories we employ to explain sets of behaviors. However, the data that we generate to come to our conclusion can help create even better results if our data are synthesized with data from others and reused. The more data we generate, and then reuse, the better our ability to resolve some of the issues stated above. Ultimately, we can paint a more accurate picture of any past event if our data are made available so that future generations of scientists can augment our past research. This is how science built upon foundation works. But this work doesn’t come without some costs.
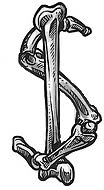
Though not fully acknowledged, we know that the data that we generate are, in fact, very valuable. From a monetary perspective, it can cost a lot of money to generate data, from lab to field equipment and labor; those who practice archaeology know that our work is a spendy endeavor. And, of course, there is the intrinsic value in the information that our research seeks to accomplish through an enhanced understanding of some past phenomena or occurrence.
Other measures by which we can measure the worth of data include:
- Its existence can save time. For example, graduate students can spend up to 80% of their time searching for data and fixing formatting issues to make it suitable for analysis. Data that’s been vetted and placed in trusted repositories can be reused by students, thus saving them a tremendous amount of time during their graduate career.
- It enables new research. Research often requires more data than one individual can collect. Thus, sharing data provides resources for future research by other teams, which should in turn advance our knowledge about a given topic.
- It allows you to get credit for data creation. Researchers who share data are more likely to be asked to be co-authors on publications using their data, or will be more frequently cited because their data are available in a trusted repository.
- Data availability establishes trust. Surveys show public trust in research is enhanced when data is available, because, unfortunately, some science has been less than trustworthy.
This last point is an important one. Regardless of our results, outcomes, and/or conclusions, the data that we generate holds onto its intrinsic and independent value, because this raw data can be reused in the scientific method.
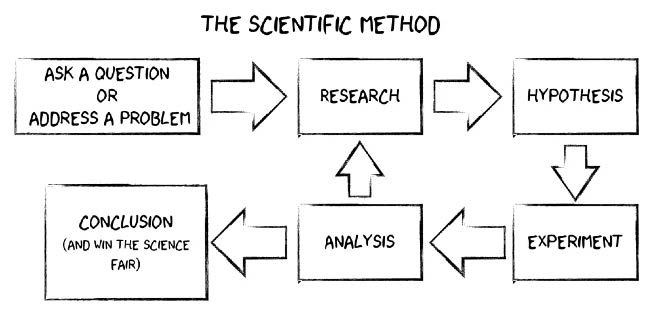
In the scientific method, the reuse of existing datasets is paramount. The methods and hypotheses we generate are built on this ideal and is why the FAIR movement (findable, accessible, interoperable, and reusable) currently has momentum across the sciences. And though FAIR is in vogue, it’s still a struggle to get scientists to share and provide data in trusted, open-access repositories for others to reuse or even evaluate.
Recently, the editor of the journal Molecular Brain, Tsuyoshi Miyakawa, penned an editorial entitled “No raw data, no science: another possible source of the reproducibility crisis”, a commentary on data availability in peer-review. He writes, “….97% of the 41 manuscripts did not present the raw data supporting their results when requested by an editor, suggesting a possibility that the raw data did not exist from the beginning, at least in some portions of these cases.” So not only is there no metadata that describes what the data are, but the data themselves did not exist? That’s really hard to stomach, but it seems to be an unfortunate truth. And Miyakawa is not alone in his observations.
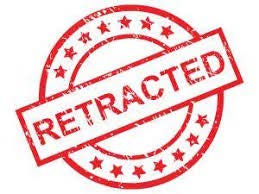
The editors of the Lancet, one of the most prestigious journals in medicine (impact factor = 59.102 versus Journal of Archaeological Science impact factor = 3.030) recently retracted a very high-profile article titled “Hydroxychloroquine or chloroquine with or without a macrolide for treatment of COVID-19: a multinational registry analysis”. The authors claimed to use an international database of patient data created by the company Surgisphere Corporation to evaluate the efficacy of Hydroxychloroquine in treating Covid-19. Their results are notable and resulted in the WHO recommending the discontinuation of this as a treatment. However, this may have been done in haste. It turns out Surgisphere “declined to make the underlying data…available for an independent audit”, calling into question the article’s results. It’s entirely possible that their data did not really exist. How is it even possible that this type of academic fraud occurs in today’s science community? Will we ever be able to truly say that science abides by the FAIR principles when there are academics and journals that don’t hold scientists and their data accountable like we do of their interpretations of data?
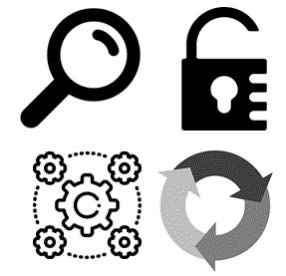
As someone who works for a Center whose mission it is to archive, preserve, and make data available, I hope that the archaeology community begins to embrace the idea that the quality and availability of their data are just as important as the original interpretations of that data. There is good science and bad science. Good science, and scientists, make data available. This enables others to reproduce and corroborate, or even dispute, conclusions drawn in a study. That’s how real science is intended to work.
Findable, accessible, interoperable, and reusable/reproducible data are the foundation of good science, and are more important than our interpretations. Our ability to serve as trusted scientists lies not only in our ability to push the frontiers of knowledge, but also in our willingness to be transparent and accountable about our data. In this way, our conclusions – while no doubt thought-provoking – pale in comparison to the manner in which we generate and contribute data. This paradigm shift is paramount for good science to flourish; and the first step is letting our data loose to be reused and productive in someone else’s hands.